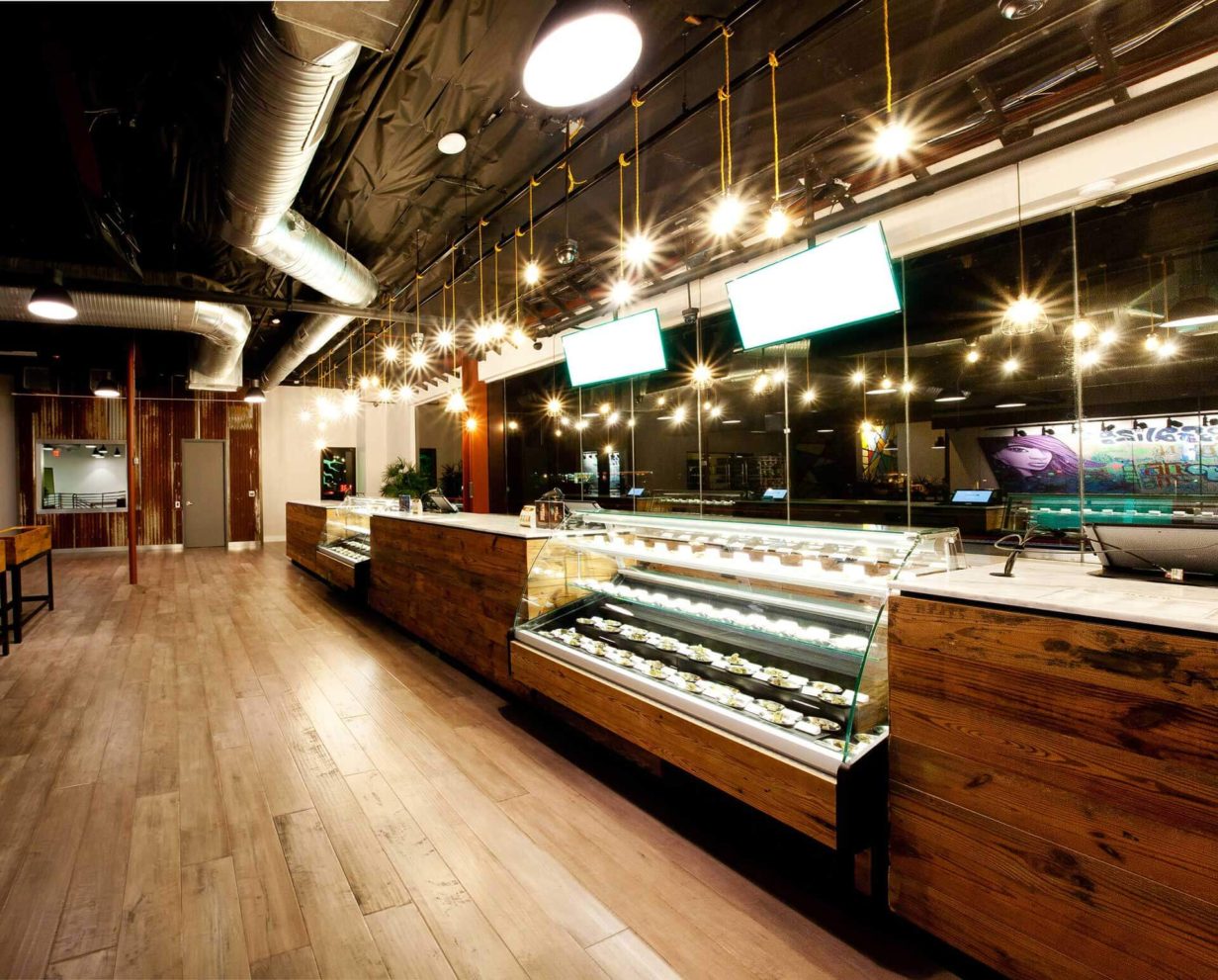
In-Store Trends and Segmentation of Cannabis Consumers via Factor Analysis
An original database was constructed using output from a retail POS Software. Methods used produced a database capable of analysis currently unavailable using the POS interface. The final database contained 523,724 transactions and span a period between 01 March 2018 -to- 09 August 2010 (75 weeks).
To perform the analysis 3 available databases were used. A customer database, an invoice report and an itemized sales report. The invoice report was merged with the itemized sales report so that names could be associated with product purchases. The customer report was then merged with the combined dataset so that ages and ZIP codes could also be associated with product purchases. In order to complete the analysis, modifications were made to the dataset so that the final data contained only observations associated with an identifiable and unique person characterized by a first name, last name, birth-date, city and ZIP.
All customer records with duplicate names and missing data were dropped. The dataset was restricted to only those entries with complete records. As the POS software is unable to provide purchase records associated with individuals and the data contained in the invoice report only contains names, any customers with duplicate names had to be removed from the database. Code was developed to manage the merges and extraction of dependable records so that in the future the master database can be reconstructed via automated processes.
A factor analysis was used to describe variability among observed, correlated variables in the dataset, in order to identify whether a number of unknown segments would emerge. Upon review of the results, 5 potential segments could be identified. 90,322 active customers were then grouped into 1 of the 5 segments based on predicted values. Groups of similar customer size emerged from the data which could be categorized as psychographic segments, based on purchase behavior and product preferences. Geodemographic segmentation was used to identify top performing ZIP codes as well as how much of the total segments they represent, while also providing important clues about the group.